Internet advertisers and the National Security Agency are not the only ones dealing with the “data deluge” lately. Scientists, too, have access to unprecedented amounts of data, both historical and real-time data from around the world. For instance, using sensors located on ocean buoys and the ocean floor, oceanographers at the National Oceanographic and Atmospheric Administration have modeled tsunamis in real-time immediately after the detection of large earthquakes. This technology was used to provide important information shortly after the 2011 Tohoku earthquake off the coast of Japan.
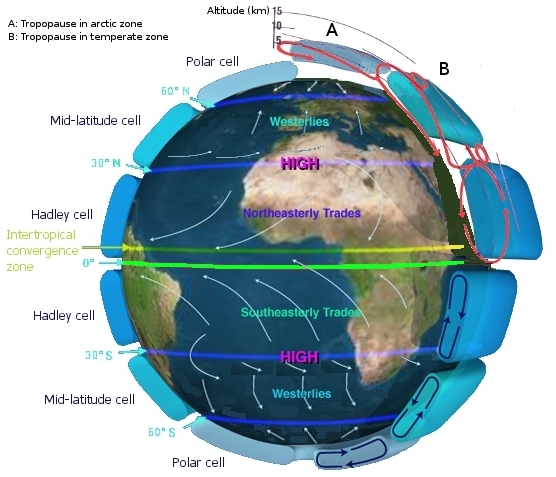
The process of incorporating data into models is a nontrivial task and represents a large research area within the fields of weather and climate modeling. While much of this data may be real-time observations, climate scientists also deal with a significant amount of historical data, such as oxygen isotope ratios measured from glacier ice cores. A major question facing climate modelers is how best to incorporate such data into models. As climate models increase in complexity, their results become correspondingly more intricate. Such models represent climate processes spanning multiple spatial and temporal scales and must relate disparate physical phenomena. Data assimilation (DA) is a technique used to combine observations with model forecasts in order optimize model prediction. DA has many potential useful applications in climate modeling. Combining observational data with models is a mathematical issue which can manifest itself in many areas, including model parametrization, model initialization, and validation of the model prediction against observations. For instance, the continued improvement of weather and storm surge models can be attributed in large part to successful parameterizations and DA, in addition to greater computing power.
This week’s Hot Topics Workshop on “Predictability in Earth System Processes” at the Institute for Mathematics and Its Applications (IMA) at the University of Minnesota (November 18-21, 2013) will identify challenges to assimilating data in climate processes, while highlighting mathematical tools used to approach these problems in general. Specific goals of the workshop are to identify DA problems in climate modeling, and to investigate new mathematical approaches to open problems such as:
- Improvement of weather forecasts by increasing accuracy to periods of several weeks to months through effective use of DA;
- Effectively apply uncertainty quantification in predictions from Earth system models arising from model errors and observational errors using DA; and
- Implementation of topological data analysis techniques to provide insight into the state space of the model.
This is an exciting workshop, as one of its primary aims is to bring together scientists and mathematicians from a wide range of subdisciplines whose paths might not typically intersect. We anticipate substantial cross-pollination of ideas at this workshop and expect to identify scientific challenges and form interdisciplinary collaborations in order to address a number of mathematical issues in climate research.
Jesse Berwald
On behalf of the Organizers
Thomas Bellsky Arizona State University
Jesse Berwald University of Minnesota, Twin Cities
Lewis Mitchell University of Vermont